What will British healthcare look like in 20 years' time?
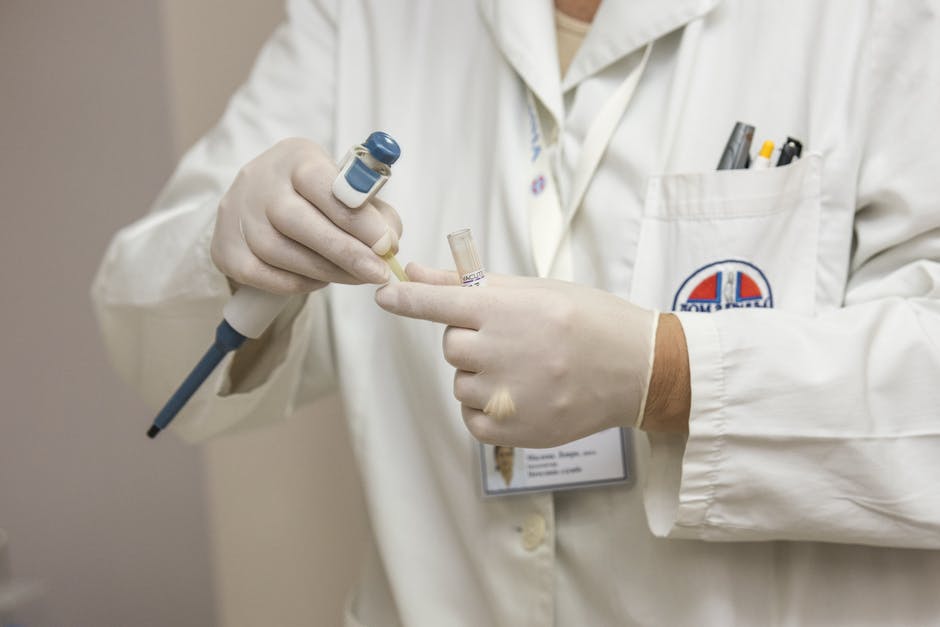
In today’s healthcare system, the quantity of data is growing exponentially. Hospitals will follow GP practices by universally adopting Electronic Healthcare Records (EHR) systems [1]; it will not be long before patients are using wearable (or even implantable) technology with continuous monitoring; and human genome sequencing is becoming ever cheaper. This essay provides a speculative but detailed view of how this data can be used to improve the treatment of chronic disease using Artificial Intelligence (AI) over the next 20 years.
A clinical decision support system (CDSS) is an application that analyses data to help healthcare providers make clinical decisions. CDSSs based on decision trees (a form of machine learning) have already been implemented e.g. NHS Pathways; a triage system for NHS 111 and 999 calls. CDSSs have also been integrated with EHR software, where they produce reminder boxes for patient follow-up, warning systems for drug interactions and diagnostic suggestions. A systematic review concluded that they improved practitioner performance by 64% and patient outcomes by 13% [2]. Nevertheless, most hospitals in the UK do not use any form of CDSS.
At the same time, the ageing population presents an increasing need for intelligent CDSSs. Comorbidity is becoming increasingly common [3]; and health conditions that affect the elderly are typically chronic and difficult to treat [4]. There is huge scope to reduce medical errors and financial costs. It has been reported that up to 35% of outpatients and 40% of hospitalised elderly experience an adverse drug reaction, half of which are preventable [5]. Moreover, a survey of 225 clinicians [6] showed willingness to accept decision support when the patient is elderly, has multiple medications or chronic conditions.
CDSSs will be able to reduce clinicians’ workload by taking over certain tasks. Once technology has superceeded humans in diagnostics and patient management, it will give clinicians more time for things that a computer can’t achieve in 20 years: empathetic communication, clinical examination, practical procedures etc.
However, this can’t happen unless a new form of CDSS is introduced. Systems based on decision trees cannot cope with the complexity of handling long decision sequences, such as the treatment of chronic disorders. Reinforcement Learning (RL) is an AI method with far greater flexibility. It works on the principle of receiving quantitative feedback while interacting with the environment. Readers with a biological background may find the analogy with Pavlovian Conditioning helpful i.e. you touch a hot pan and you burn yourself… you learn not to touch it again! RL is based on the same learning principles as humans, meaning that it has great potential for lifelong learning within medicine. Despite it’s applicability to medicine, the literature is relatively sparse compared to other applied disciplines, such as gaming and robotics [7][8][9]. In 20 years time, I envisage a fully integrated CDSS-EHR system that is able to assimilate experience over time and adapt to the needs of individual patients.
There are three crucial steps in developing this methodology:
- Development of a realistic simulation for exploring healthcare policies
- Compatibility with EHR software
- Acceptance in the medical community
All three of these steps will be challenging. Firstly, the simulation; why is it needed? Most RL research is conducted on games rather than real world problems e.g. the famous AlphaGo [10]. In medicine, trial-and-error learning will require a simulated environment to avoid unsafe experimenting on patients! There is an open source platform; Observational Medical Dataset Simulator [11], that creates a realistic simulation based on EHRs.
Compatibility can be achieved using the Fast Healthcare Interoperability Resources Application Programming Interface (FHIR API). FHIR API is free and has recently been adopted by DeepMind Health for their Streams application [12]. It is supported by NHS Digital, NHS England and the INTEROPen group.
Finally, acceptance in the medical community will be a major obstacle. Intelligent CDSSs are “black boxes”; clinicians don’t understand how they work and they will resist their implementation. This should be met with sensitivity and measures can be taken to expedite acceptance. For example, the CDSS should be able to communicate its reasoning by quantifying the evidence for it’s treatment recommendations. Clinicians are held accountable by law and by the GMC; they can only carry out a recommendation if they can justify that decision to themselves. It is unlikely that doctors will be able to interpret the calculations carried out by the CDSS. However, a possible solution is to predict the consequences of each intervention over time (with confidence statistics) and present the information in a way that is understandable. Clinicians’ may appreciate a searching algorithm to identify similar historical cases to back up these predictions.
In summary, there is great potential for AI in medicine, provided that clinicians and computer scientists are willing to collaborate. The sooner this happens the better; the current state of the NHS is unsustainable and AI could be the solution.
References
[1] Albert Boonstra, Arie Versluis and Janita F. J. Vos. “Implementing electronic health records in hospitals: a systematic literature review.” BMC health services research 14.1 (2014), p. 370.
[2] Amit X. Garg et al. “Effects of computerized clinical decision support systems on practitioner performance and patient outcomes: A systematic review”. JAMA 293.10 (2005), pp. 1223-1238.
[3] Jay F. Piccirillo et al. “The changing prevalence of comorbidity across the age spectrum”. Critical Reviews in Oncology/Hematology 67.2 (2008), pp. 124-132.
[4] Robert L. Maher Jr, Joseph T. Hanlon and Emily R. Hajjar. “Clinical consequences of polypharmacy en elderly”. Expert opinion on drug safety 13.1 (2014), pp. 1-11.
[5] Katja M. Hakkarainen et al. “Percentage of patients with preventable adverse drug reactions and preventability of adverse drug reactions – a meta-analysis”. PLoS ONE 7.3 (2012), pp. 11-13.
[6] Dean F. Sittig et al. “A survey of factors affecting clinician acceptance of clinical decision support”. BMC Medical Informatics and Decision Making 6.1 (2006), p. 6.
[7] Liliana Orellana, Andrea Rotnitzky and James M Robins. “Dynamic Regime Marginal Structural Mean Models for Estimation of Optimal Dynamic Treatment Regimes, Part II: Proofs of Results.” The international journal of biostatistics 6.2 (2010), Article 9.
[8] Mary L. Mcmaster et al. “Dynamic Treatment Regimes”. Clinical Lymphoma 9.1 (2010), pp. 19-22.
[9] Susan M. Shortreed et al. “Informing sequential clinical decision-making through reinforcement learning: an empirical study”. Machine Learning 84 (2011), pp. 109-136.
[10] David Silver et al. “Mastering the game of Go with deep neural networks and tree search”. Nature 529.7587 (2016), pp. 484-489.
[11] Richard E. Murray, Patrick B. Ryan and Stephanie J. Reisinger. “Design and validation of a data simulation model for longitudinal healthcare data”. AMIA Annu Symp Proc (2011), pp. 1176-1185.
[12] DeepMind. Streaming the right information to the right clinician at the right time. 2017. url: www.deepmind.com/applied/deepmind- health/ streams (visited on 22/04/2017).
Article photo credit: Emiliano Ricci
- Log in to post comments