“Good morning, the computer will see you now.”
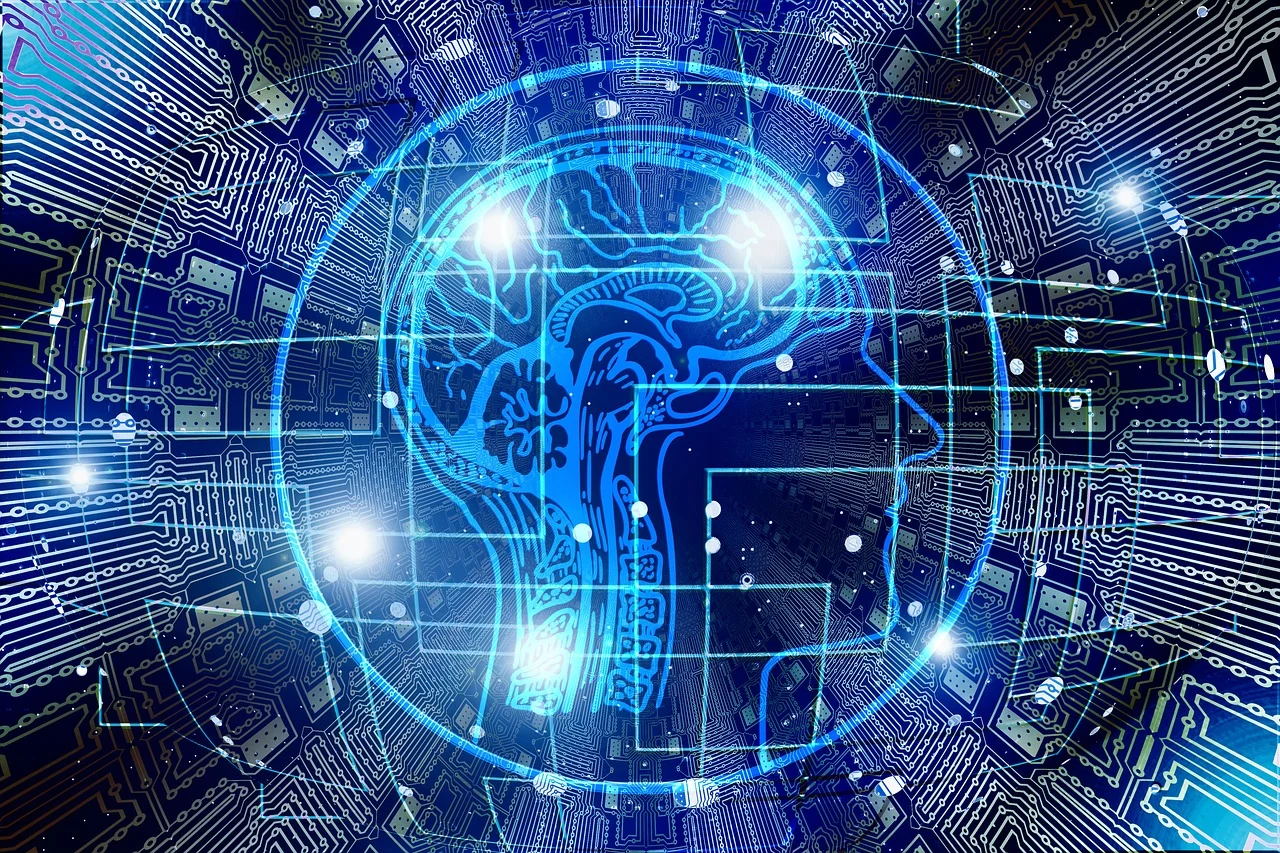
Imagine that you have a chesty cough that has been plaguing you for ten days or so. You find this cough concerning, and so you book an appointment with your GP service. Now, imagine that you arrive at your local clinic and are given two options: your particular constellation of symptoms can be addressed by a living, breathing doctor – who may or may not be a taxonomist of coughs, with years of practice differentiating the rales of congestive heart failure from phlegmy pneumonias – or by a computer. Specifically, by an 'inference engine', a formidable set of machine learning systems, capable of analysing hundreds of billions of combinations of risk factors, symptoms and diseases within seconds [1] and arriving at a probable diagnosis. Twenty years ago, this scenario might have seemed like an extract from an ambitious sci-fi movie, but in 2019, companies such as Babylon Health suggest that it will soon be commonplace [1].
Indeed, artificial intelligence (AI)-based methods – such as machine learning systems – have emerged as powerful tools to be wielded in the medical sector, with some arguing that AI is already transforming healthcare [2]. From the outset, the foundations of assessing this claim are built upon understanding what is meant exactly by 'AI', and which instruments form part of its arsenal. AI is broadly defined as the simulation of human intelligence processes by machines, especially computer systems. Key aspects of cognition to be replicated include the ability to acquire information and rules for using it (learning), reasoning skills (using rules to reach conclusions), and the capacity for self-correction [3].
AI devices are considered to fall mainly into two major categories: the aforementioned machine learning (ML) techniques and natural language processing (NLP) methods [4]. In medicine, ML techniques are used to analyse structured data – i.e., data that have been organised based on their characteristics – such as diagnostic imaging, genetic data and electrophysiological data. ML procedures can, for example, cluster patients' traits, and use them to suggest diagnoses or make personalised recommendations of drugs to be prescribed [5]. By contrast, NLP methods extract information from unstructured data, such as human speech or unorganised texts – potentially even the illegible scribbles of clinicians – such that the computer can understand, and then use, every-day human language [1, 4]. These technologies do not operate in a binary fashion; rather, they are 'symbiotic': ML can teach NLP systems new languages, whilst NLP procedures can turn texts into machine-readable structured data, which can then be analysed by ML techniques.
Given that the central function of AI technologies is the analysis of data, and that healthcare is an intrinsically data-heavy field, it is unsurprising that medicine has become a prime candidate for the application of AI. From establishing diagnoses to systematising drug discovery and uncovering hidden trends in epidemiology, the permeation of AI into a multitude of medical disciplines is evidenced by an increasingly rich research literature. Arguably, the most advanced use of AI as yet is in diagnostics. Esteva et al. reported in 2017 that 'deep learning' – a spin-off of ML, making use of more sophisticated algorithms – could be used to achieve a "dermatologist-level" of skin cancer classification [6]. More recently, Liang et al. have provided evidence that AI used in paediatrics can have a diagnostic accuracy "comparable to experienced paediatricians [7]". In brief, it is a converging – and growingly evidence-based – belief that AI may well be an equal-match for the MD, at least in working out which pathology is ailing a patient.
Herein lies one of the first concerns expressed by many with respect to the infiltration of AI into healthcare: will it make doctors redundant? The answer is that it's unlikely. It is rightly instilled in us as medical students that we are more than pattern detectors, and drug prescribers. AI may be trained to be as successful as any doctor in these practices, but is unlikely to be able to place a chest drain into Ms. Y, or to hold Mr. X's hand as it discusses with him options for end-of-life care. Clinical practice often involves complex behaviours, such as the ability to read social cues and to utilise contextual knowledge, that cannot – as yet – be learnt by AI, and which may even be tacit, and so unteachable. Overall, there are a number of reasons why I might have been smarter to choose a career in the City rather than in medicine, but the risk that a computer might eventually take my job is not particularly plausible as one of them.
Rather, it may be more useful to consider how physician and computer might complement each other. AI could be used rationally to provide further supporting evidence for a human (ideally, a medic) to make a diagnosis, and to suggest treatment options that might be suitable for particular patients, rather than to decide between them and print a prescription. Further, AI may actually expand the treatment options that are available to patients. In the field of mental health, for instance, budgets have failed to match other areas of the NHS [8], leaving services such as cognitive behavioural therapy (CBT) frequently oversubscribed. AI could be, and in some cases is being, harnessed by smartphone apps that can analyse people's symptoms – usually self-reported using a chatbot – and spot patterns before offering advice to patients or healthcare workers on what action to take. For example, 'Woebot' is a widely available AI "therapy chatbot" trained in the principles of CBT that has been deemed "surprisingly helpful" by reviewers [9]. Given the enduring practical limitations – largely, problems of funding and staffing – that leave NHS mental providers often unable to meet demand, AI-based treatments may prove a useful adjunct to services.
Whilst AI technologies are attracting significant attention – and generating excitement – in medical research, their real-life implementation faces obstacles that are likely to be long-standing – particularly, if that 'implementation' is to occur within the context of the NHS. The first hurdle comes from AI's own limits: significant computing power is required for the analysis of large and complex data sets, and all the data also need to be digitised. Investing sufficient resources from an NHS that seems to suffer from 'chronically stretched syndrome' into AI equipment is likely to pose a problem. This is abetted by the fact that medical data are not consistently digitised across the NHS, and even when they are, there is a lack of standardisation in NHS IT systems that might still constrain AI's utility [10].
Ethically, there are also questions about the extent to which patients and doctors are comfortable with the digital sharing of personal health data, and how the security of that data may be protected. After all, medical data are not just voluminous, but also sensitive: at the other end of lists of blood test results, chest x-rays, and case descriptions are human beings with the "right to respect for private life," only violable in the interests of "the protection of the public health [11]". This does not include, for example, a security breach, or the sharing of data without consent, even if it is to develop AI technologies. Indeed, it was only in 2017 that the U.K.'s data protection regulator ruled that the Royal Free Hospital was wrong to share details of 1.6 million patients with Google's AI company DeepMind [12], even if the data were intended to be used to improve diagnostic services at the hospital.
Overall, it would appear that the clinical benefits that AI proposes are inextricable from vital concerns about transparency, safety and accountability. Whilst the U.K. has implemented a "Code of conduct for data-driven health and care technology" to outline how data may be used appropriately [13], it is likely that further regulations will be needed, providing (a) formal standards to assess the safety and efficacy of AI systems, and (b) more specific guidelines of usage, corresponding with the particular AI system(s) to be adopted.
Even if all the latter were achieved, other social issues would remain. For example, AI systems could be biased by the data used to train them, and hence make unfair decisions that reflect wider prejudices in society. Dealing with such problems is likely to prove even more difficult than regulating the usage of AI, since it would involve being honest about issues such as racism, classism and all other manner of '-isms' that permeate our society and, frankly, no country likes to do that.
Nonetheless, it is an inevitable reality that, slowly but surely, AI is percolating into almost every aspect of our lives. It is already busy in the background of routine tasks, powering virtual assistants like Siri and Alexa, recommendations from Netflix, and underpinning billions of Google searches each day. It would be naïve to believe that AI will not have profound implications for our healthcare, too. Encouragingly, a lot of research shows that AI technologies have the potential to help address important health challenges. However, it is this writer's opinion that only with sufficient regulation, societal introspection and, above all, caution, will the uptake of AI by healthcare systems be compatible with the public interest.
References
[1] AI [Internet]. Babylon Health. [cited 2019 Jul 10]. Available from: https://www.babylonhealth.com/ai
[2] PricewaterhouseCoopers. No longer science fiction, AI and robotics are transforming healthcare [Internet]. PwC. [cited 2019 Jul 10]. Available from: https://www.pwc.com/gx/en/industries/healthcare/publications/ai-robotics-new-health/transforming-healthcare.html
[3] What is AI (artificial intelligence)? - Definition from WhatIs.com [Internet]. SearchEnterpriseAI. [cited 2019 Jul 10]. Available from: https://searchenterpriseai.techtarget.com/definition/AI-Artificial-Intelligence
[4] Jiang F, Jiang Y, Zhi H, Dong Y, Li H, Ma S, et al. Artificial intelligence in healthcare: past, present and future. Stroke Vasc Neurol. 2017 Jun 21;2(4):230-43.
[5] Yelin I, Snitser O, Novich G, Katz R, Tal O, Parizade M, et al. Personal clinical history predicts antibiotic resistance of urinary tract infections. Nature Medicine. 2019 Jul;25(7):1143.
[6] Esteva A, Kuprel B, Novoa RA, Ko J, Swetter SM, Blau HM, et al. Dermatologist-level classification of skin cancer with deep neural networks. Nature. 2017 Feb;542(7639):115-8.
[7] Liang H, Tsui BY, Ni H, Valentim CCS, Baxter SL, Liu G, et al. Evaluation and accurate diagnoses of pediatric diseases using artificial intelligence. Nature Medicine. 2019 Mar;25(3):433.
[8] Funding and staffing of NHS mental health providers [Internet]. The King's Fund. 2018 [cited 2019 Jul 11]. Available from: https://www.kingsfund.org.uk/publications/funding-staffing-mental-health-providers
[9] Brodwin E. I spent 2 weeks texting a bot about my anxiety -- and found it to be surprisingly helpful [Internet]. Business Insider. [cited 2019 Jul 11]. Available from: https://www.businessinsider.com/therapy-chatbot-depression-app-what-its-like-woebot-2018-1
[10] AI in the UK: ready, willing and able? [Internet]. House of Lords Select Committee on Artificial Intelligence. 2018 [cited 2019 Jul 10]. Available from: https://publications.parliament.uk/pa/ld201719/ldselect/ldai/100/100.pdf
[11] Article 8. Human Rights Act 1998 [Internet]. [cited 2019 Jul 11]. Available from: https://www.legislation.gov.uk/ukpga/1998/42/schedule/1/part/I/chapter/7
[12] Royal Free - Google DeepMind trial failed to comply with data protection law [Internet]. 2018 [cited 2019 Jul 11]. Available from: https://ico.org.uk/about-the-ico/news-and-events/news-and-blogs/2017/07/royal-free-google-deepmind-trial-failed-to-comply-with-data-protection-law/
[13] Code of conduct for data-driven health and care technology [Internet]. GOV.UK. [cited 2019 Jul 11]. Available from: https://www.gov.uk/government/publications/code-of-conduct-for-data-driven-health-and-care-technology/initial-code-of-conduct-for-data-driven-health-and-care-technology
Article photo credit: geralt
- Log in to post comments